The Impact of AI on Credit Risk and Loan Underwriting a dramatic transformation with the advent of Artificial Intelligence (AI). AI is significantly reshaping the landscape of credit risk assessment and loan underwriting, making the process faster, more accurate, and more efficient. Traditionally, credit risk and loan underwriting were manual processes that heavily relied on human judgment and historical data, but AI is increasingly automating these functions, improving accuracy, and enabling a more nuanced understanding of risk.
This article will delve into how AI is influencing credit risk and loan underwriting. We will explore the various ways AI is being used, the benefits it offers, the challenges involved, and the future implications of AI in these crucial financial processes.
1. Understanding Credit Risk and Loan Underwriting
Before exploring the role of AI in credit risk and loan underwriting, it’s essential to define what these processes entail.
1.1. Credit Risk
Credit risk refers to the risk that a borrower may default on a loan or credit obligation. Banks and financial institutions assess this risk before approving a loan to ensure that the borrower has the ability and willingness to repay the debt. Credit risk assessment traditionally involves evaluating the borrower’s credit history, income, employment status, and other financial factors.
1.2. Loan Underwriting
Loan underwriting is the process of evaluating a borrower’s application for a loan. This includes assessing the borrower’s creditworthiness, the loan’s terms, and the likelihood that the borrower will repay the loan. Traditional underwriting methods involve a series of checks, such as credit scoring, income verification, and employment confirmation, often requiring a significant amount of time and paperwork.
2. The Role of AI in Credit Risk and Loan Underwriting
The Impact of AI on Credit Risk and Loan Underwriting, particularly through machine learning and advanced analytics, has the potential to revolutionize how financial institutions evaluate credit risk and underwrite loans. AI is capable of processing vast amounts of data at a speed and scale that humans cannot match, and it can learn from past data to identify patterns and predict future outcomes with greater accuracy.
2.1. Data-Driven Decision Making
Traditional credit risk and loan underwriting processes often rely on a limited set of data points, such as a borrower’s credit score or income level. AI, however, enables the integration of a much wider range of data sources, including:
- Alternative Data: AI can analyze alternative data sources, such as utility bill payments, rent history, and even social media activity, to get a more comprehensive view of the borrower’s financial behavior and risk profile. This is particularly useful for individuals with little or no credit history (often referred to as “credit invisibles”).
- Behavioral Data: AI systems can also track real-time consumer behavior to assess the likelihood of a borrower defaulting on a loan. By analyzing patterns in spending, saving, and paying off debts, AI models can offer a more dynamic and up-to-date risk assessment.
2.2. Machine Learning for Predictive Analytics
Machine learning, a subset of AI, is particularly useful in credit risk and loan underwriting due to its ability to predict future outcomes based on historical data. Machine learning algorithms can:
- Identify Patterns and Trends: By processing large datasets, AI systems can detect patterns in borrower behavior that may indicate future credit risk. For example, AI models can identify borrowers who may be more likely to default on loans based on their past financial behavior, even before they exhibit overt signs of financial distress.
- Refine Credit Scoring Models: AI can enhance traditional credit scoring models (e.g., FICO score) by incorporating a wider range of data and adjusting risk factors based on real-time data. This results in a more accurate and holistic credit score that better reflects the borrower’s current financial situation.
2.3. Automation of the Underwriting Process
AI is increasingly automating various aspects of the loan underwriting process, reducing the need for manual intervention and accelerating decision-making.
- Automated Document Verification: AI-powered systems can automatically verify the accuracy of documents submitted by borrowers, such as income statements, tax returns, and bank statements. Optical character recognition (OCR) and natural language processing (NLP) technologies can extract data from documents quickly and accurately, reducing errors and speeding up the underwriting process.
- Instant Decision-Making: AI models can process data and make real-time decisions about whether to approve or decline a loan application, bypassing the need for manual review by underwriters. This instant decision-making improves the customer experience by providing faster loan approvals and denials.
3. Benefits of AI in Credit Risk and Loan Underwriting
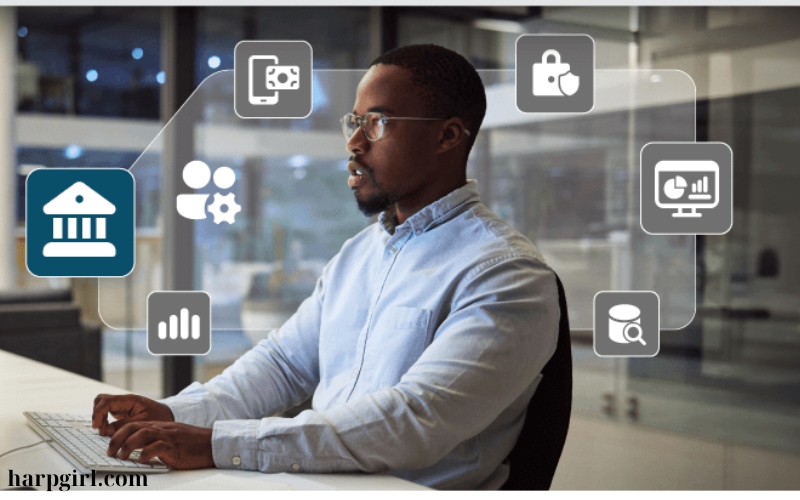
The Impact of AI on Credit Risk and Loan Underwriting in credit risk and loan underwriting offers several significant advantages for both financial institutions and borrowers.
3.1. Enhanced Accuracy and Reduced Human Bias
The Impact of AI on Credit Risk and Loan Underwriting of AI in these areas is the reduction of human error and bias. Traditional underwriting processes often involve subjective decision-making, where underwriters may unintentionally allow personal biases to affect their judgment. AI, on the other hand, uses data-driven algorithms that focus purely on objective information, leading to more accurate assessments of risk.
- Minimizing Bias: AI models, when properly trained, can eliminate biases based on factors such as gender, race, or age, which may influence human decision-making in the underwriting process. This creates a more fair and equitable lending process.
- More Accurate Risk Assessment: By analyzing a broader range of data and learning from past cases, AI is better equipped to make precise predictions about a borrower’s creditworthiness. As a result, financial institutions are more likely to approve loans to creditworthy borrowers and decline high-risk applicants.
3.2. Faster Decision-Making and Improved Efficiency
AI-powered systems can process vast amounts of data quickly, enabling faster credit risk assessments and loan underwriting decisions. This speed is crucial for enhancing the customer experience and increasing operational efficiency.
- Instant Loan Approvals: AI can provide instant credit decisions, reducing the time it takes for borrowers to receive a loan approval or rejection. This is particularly important in today’s fast-paced, digital-first economy, where customers expect quick responses.
- Cost Reduction: Automation of the underwriting process reduces the need for human intervention, lowering operational costs for financial institutions. This allows lenders to focus their resources on more complex cases while streamlining the handling of routine applications.
3.3. Improved Customer Experience
AI can improve the overall customer experience by offering a more personalized and efficient loan application process.
- Tailored Loan Products: By analyzing a borrower’s financial data, AI can recommend the most suitable loan products, terms, and interest rates for individual customers. This personalized approach enhances customer satisfaction and can help increase loan approval rates.
- 24/7 Availability: AI-powered loan platforms are available around the clock, allowing customers to apply for loans and receive decisions at any time. This level of accessibility and convenience is a significant improvement over traditional, branch-based lending systems.
4. Challenges of AI in Credit Risk and Loan Underwriting
While AI offers significant benefits, its integration into credit risk assessment and loan underwriting also presents several challenges.
4.1. Data Privacy and Security Concerns
AI relies heavily on vast amounts of data, including sensitive personal and financial information. Ensuring that this data is protected and used responsibly is critical for maintaining consumer trust and complying with data privacy regulations, such as the General Data Protection Regulation (GDPR) in Europe or the California Consumer Privacy Act (CCPA) in the United States.
- Data Protection: Financial institutions must implement strong cybersecurity measures to protect borrower data from breaches, as any compromise of this data can have severe consequences.
- Ethical Use of Data: There is also the issue of ensuring that AI models are used ethically, with transparency regarding how consumer data is utilized and how credit decisions are made.
4.2. Algorithmic Transparency and Accountability
AI models, particularly machine learning algorithms, can sometimes be seen as “black boxes” where the decision-making process is not entirely transparent. This lack of transparency can raise concerns about fairness, especially when applicants are denied loans without a clear explanation.
- Regulatory Scrutiny: As AI becomes more prevalent in lending decisions, regulators are increasingly focused on ensuring that AI-driven credit assessments are fair, unbiased, and transparent. Financial institutions must be able to explain the rationale behind AI-driven decisions to meet regulatory requirements and avoid potential legal challenges.
- Bias in AI Models: While AI has the potential to reduce human bias, it is still possible for AI models to inherit biases from the data they are trained on. If the training data contains biases (e.g., historical discrimination against certain groups), the AI model may perpetuate these biases, leading to unfair lending decisions.
4.3. Integration with Legacy Systems
Many financial institutions still rely on legacy systems for their credit risk and loan underwriting processes. Integrating AI with these outdated systems can be complex and costly, requiring significant investment in technology upgrades and training.
- System Compatibility: AI-powered solutions must be compatible with existing IT infrastructure, which may require substantial customization and integration work.
- Change Management: Successfully adopting AI in credit risk and loan underwriting involves managing the cultural and organizational changes associated with automation, including upskilling staff and ensuring smooth collaboration between AI tools and human decision-makers.
5. The Future of AI in Credit Risk and Loan Underwriting
The future of AI in credit risk and loan underwriting is promising, with continued advancements in AI technologies likely to further improve the accuracy, efficiency, and fairness of these processes. Key developments to look out for include:
- Explainable AI (XAI): As concerns about the transparency of AI models grow, there will be a push towards more explainable AI that can provide clear, understandable justifications for its decisions.
- AI-Powered Credit Scoring: The integration of AI with alternative data sources could lead to the development of more sophisticated credit scoring models, improving access to credit for underserved populations.
- Blockchain and AI Integration: Combining AI with blockchain technology could enhance the security, transparency, and efficiency of loan underwriting processes, enabling faster, more secure transactions.