How Data Analytics is Revolutionizing, the financial services industry has experienced a radical transformation, driven largely by the advent of digital technologies. One of the most profound shifts has been in customer service. Banks are increasingly relying on data analytics to enhance their services, improve customer satisfaction, and streamline operations. Data analytics provides invaluable insights into customer behavior, preferences, and needs, enabling banks to offer more personalized, responsive, and efficient services. The integration of data analytics into customer service operations is not just a trend, but a necessary evolution to stay competitive in an increasingly digital and customer-centric market.
This article explores how data analytics is revolutionizing customer service in banking, with a focus on key technologies, benefits, challenges, and future trends. From predictive analytics to real-time customer support, data is playing a pivotal role in shaping the future of banking interactions.
1. Understanding Data Analytics in Banking
Data analytics in banking refers to the process of analyzing large sets of financial data and customer information to extract meaningful insights that can improve decision-making, service delivery, and overall customer experience. The insights derived from data analytics help banks to better understand their customers, predict future behaviors, and optimize their operations.
1.1. The Role of Big Data and Artificial Intelligence (AI)
- Big Data: With the growing volume of transactions, social media interactions, and customer interactions, banks now collect an enormous amount of data. Big data tools allow banks to analyze this vast amount of information in real time, extracting patterns and trends that were previously undetectable.
- Artificial Intelligence: AI plays a crucial role in data analytics by enabling machines to process vast quantities of data and learn from it. Machine learning (ML) algorithms can predict customer behavior, automate customer service tasks, and even anticipate potential issues before they arise.
2. How Data Analytics is Enhancing Customer Service
The application of data analytics in customer service is transforming how banks interact with clients, creating a more seamless and personalized experience. Below are the major ways data analytics is enhancing customer service in banking.
2.1. Personalization of Banking Services
One of the most significant impacts of data analytics is the ability to offer highly personalized services to customers. Through the analysis of transactional data, demographic information, and behavior patterns, banks can tailor their offerings to meet individual needs.
- Targeted Product Recommendations: By analyzing a customer’s past behavior, transaction history, and financial goals, banks can offer personalized product recommendations such as loans, investment opportunities, or credit cards. This not only enhances the customer experience but also drives revenue by promoting relevant services.
- Customized Financial Advice: Data analytics allows banks to offer customized financial advice based on an individual’s unique financial situation. Whether it’s retirement planning, budgeting, or saving for a home, data analytics helps banks provide more accurate and relevant guidance.
- Behavioral Insights: By understanding a customer’s spending habits, income patterns, and saving goals, banks can predict future financial needs and proactively offer solutions that cater to those needs.
2.2. Predictive Analytics for Proactive Customer Service
Predictive analytics allows banks to anticipate customer needs and potential problems before they occur, improving overall service and customer satisfaction.
- Customer Churn Prevention: By analyzing customer behavior and identifying early warning signs of dissatisfaction, such as changes in spending patterns or reduced engagement, banks can proactively address issues and prevent customers from leaving. This may involve offering tailored incentives, addressing customer concerns, or improving the overall user experience.
- Credit Risk Assessment: Banks can use predictive analytics to assess the likelihood of customers defaulting on loans. By analyzing a variety of factors such as income, credit history, and spending behavior, banks can make more informed lending decisions and offer personalized loan terms.
- Fraud Detection: Data analytics can help banks detect fraudulent activities in real-time by identifying unusual patterns in transaction data. By analyzing past behavior and flagging transactions that deviate from the norm, banks can prevent fraud before it impacts customers.
2.3. Chatbots and AI-Powered Customer Support
Data analytics, combined with artificial intelligence, is revolutionizing customer support in banking. AI-driven tools like chatbots and virtual assistants are becoming an essential part of customer service strategies.
- 24/7 Availability: Chatbots powered by AI can provide customers with round-the-clock assistance, answering queries, guiding them through banking processes, and helping with account management. This availability enhances customer satisfaction by ensuring help is available at any time, even outside regular banking hours.
- Natural Language Processing (NLP): NLP allows AI systems to understand and process human language, enabling chatbots to hold more sophisticated and natural conversations with customers. This means that customers can receive personalized and context-aware responses, which can solve their problems more efficiently.
- Efficient Issue Resolution: Chatbots can handle a wide range of customer service requests, from checking account balances to processing payments, without requiring human intervention. This frees up bank employees to focus on more complex issues, improving overall efficiency and reducing wait times for customers.
2.4. Real-Time Customer Service and Support
Data analytics enables banks to offer real-time customer service, improving the responsiveness of customer support teams. By leveraging data in real-time, banks can provide quicker solutions to customer problems and improve overall satisfaction.
- Omnichannel Support: Data analytics helps banks provide seamless customer service across various channels—whether it’s through a mobile app, website, phone support, or in-person at a branch. By integrating data from all these touchpoints, banks can offer a consistent and personalized experience regardless of how the customer chooses to interact.
- Quick Resolution of Customer Issues: With real-time data, customer service representatives can quickly access a customer’s account information, history, and previous interactions. This allows them to resolve issues more efficiently without needing to ask customers to repeat their information or wait for further investigation.
3. Operational Efficiency Through Data Analytics
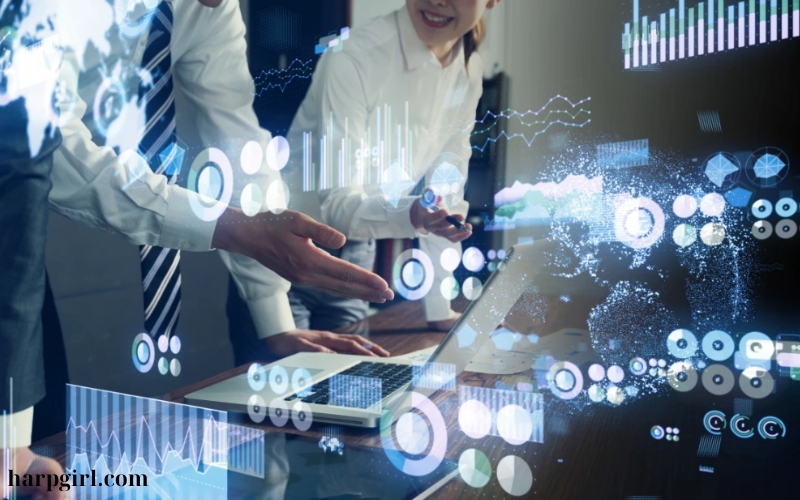
Data analytics does not only improve customer-facing interactions; it also enhances the operational efficiency of banking institutions. By optimizing internal processes, banks can offer a more streamlined and cost-effective customer service experience.
3.1. Automation of Routine Tasks
By automating routine tasks, such as account management, loan approvals, and transaction processing, data analytics helps banks reduce human error and operational costs while speeding up service delivery.
- Loan Approval Automation: Machine learning algorithms can analyze vast amounts of financial data to automate loan approval processes. This not only reduces the time taken to process applications but also ensures more consistent and objective decision-making.
- Document Verification: Data analytics can also automate document verification for new customers or loan applicants, reducing the time required for manual review and improving accuracy.
3.2. Cost Reduction
How Data Analytics is Revolutionizing costs by optimizing processes, leveraging self-service technologies, and improving workflow efficiency through data analytics.
- Cost-Effective Customer Support: By using chatbots, automated responses, and AI-powered tools, banks can reduce the need for large customer support teams while still delivering high-quality service.
- Resource Allocation: Data analytics helps banks allocate resources more effectively by analyzing customer service data to identify peak hours, common queries, and areas for improvement. This enables better staffing decisions and reduces costs associated with overstaffing or underperforming areas.
4. Challenges in Implementing Data Analytics in Customer Service
While data analytics holds immense potential for improving customer service in banking, there are several challenges that banks must address in its implementation.
4.1. Data Privacy and Security
With the increasing use of customer data comes the responsibility to protect that data. Banks must ensure that they comply with stringent data privacy regulations, such as the General Data Protection Regulation (GDPR), to maintain customer trust.
- Secure Data Management: Data analytics involves collecting, processing, and storing vast amounts of sensitive customer information. Banks must implement robust cybersecurity measures to protect this data from breaches and cyberattacks.
- Transparency and Consent: Customers must be informed about how their data will be used and have the ability to opt-out or limit the sharing of their information.
4.2. Data Quality and Integration
For data analytics to be effective, banks must ensure the quality and accuracy of the data they collect. Inconsistent, incomplete, or inaccurate data can lead to incorrect insights and undermine customer service efforts.
- Data Integration: Banks must integrate data from multiple sources, including transaction records, customer interactions, and social media channels, to build a comprehensive view of each customer. This requires the development of sophisticated data management systems.
- Data Cleansing: Regular data cleaning processes are necessary to maintain the accuracy and relevance of the data used in analytics.
4.3. Customer Trust in AI-Driven Services
As banks increasingly rely on AI and machine learning for customer service, there is a risk that customers may feel uncomfortable with automated services or lose trust in the technology.
- Human Element: While AI can enhance customer service, banks must strike a balance between automation and human interaction. Many customers still prefer human contact, especially for complex or emotionally sensitive issues.
5. The Future of Data Analytics in Banking
The future of data analytics in banking is bright, with several exciting possibilities on the horizon. As technology continues to advance, banks will find new ways to use data analytics to further enhance customer service.
5.1. Advanced Predictive Analytics
As machine learning algorithms improve, banks will be able to predict customer needs and preferences with even greater accuracy. This will enable hyper-personalization of banking services, where customers receive offers, recommendations, and services tailored to their exact financial situation and goals.
5.2. Real-Time Customer Insights
Banks will increasingly use real-time data to improve customer interactions. The ability to respond to customer inquiries, solve problems, and offer solutions in real-time will become the norm, creating a seamless customer service experience.
5.3. Voice and Biometric Recognition
The future of data analytics in customer service may include voice recognition and biometric authentication as key tools for improving the customer experience. Voice-activated banking, driven by AI and natural language processing, could further streamline customer service, allowing for even greater convenience and personalization.