Artificial Intelligence and Machine Learning and Machine Learning (ML) are transforming the world at an unprecedented pace, revolutionizing industries, reshaping economies, and influencing the way we live and work. As technological advancements continue to push boundaries, AI and ML are playing a central role in developing smarter systems, enhancing decision-making processes, and improving overall productivity. This article explores the potential of AI and ML, how they are shaping various sectors, and what the future holds in terms of their impact on society, the economy, and everyday life.
1. Introduction: The Rise of Artificial Intelligence and Machine Learning
Artificial Intelligence, the field that focuses on creating systems capable of performing tasks that would typically require human intelligence, encompasses a wide range of technologies. From speech recognition to natural language processing (NLP), AI has found applications across a spectrum of industries, including healthcare, finance, retail, and manufacturing.
Machine Learning, a subset of AI, involves the development of algorithms and statistical models that allow systems to “learn” from data and make predictions or decisions without being explicitly programmed. Through the analysis of large datasets, ML algorithms identify patterns, make informed decisions, and continuously improve over time.
The synergy between AI and ML is ushering in a new era of automation, efficiency, and innovation, positioning these technologies as key drivers of future progress.
2. Key Components and Technologies Behind AI and ML
2.1. Natural Language Processing (NLP)
Natural Language Processing enables machines to understand, interpret, and respond to human language in a way that is both meaningful and contextually appropriate. NLP powers various applications, from chatbots and virtual assistants to translation services and sentiment analysis.
The growing sophistication of NLP models, such as OpenAI’s GPT (like the one used in this conversation), has made it possible for machines to engage in more fluid, human-like conversations, breaking down communication barriers and enhancing user experiences across industries.
2.2. Deep Learning
Deep learning, a subfield of ML, uses neural networks with many layers to model complex patterns in large datasets. Inspired by the human brain’s structure, deep learning algorithms have proven to be particularly effective in tasks such as image recognition, speech recognition, and autonomous driving.
Deep learning has significantly advanced AI applications, with systems capable of learning from vast amounts of unstructured data, such as images, videos, and text. This enables AI to outperform traditional machine learning models in a variety of tasks, particularly in the realm of artificial vision and language understanding.
2.3. Reinforcement Learning
Artificial Intelligence and Machine Learning is an area of ML where agents are trained to make decisions by interacting with their environment. Instead of being provided with explicit examples, the system learns through trial and error, receiving rewards or penalties based on the actions it takes.
RL is essential in fields such as robotics and game playing (e.g., AlphaGo), where systems need to develop strategies and improve their decision-making processes over time. This type of learning allows AI to adapt to dynamic environments and make autonomous decisions with little human intervention.
3. AI and ML in Industry: Revolutionizing Key Sectors
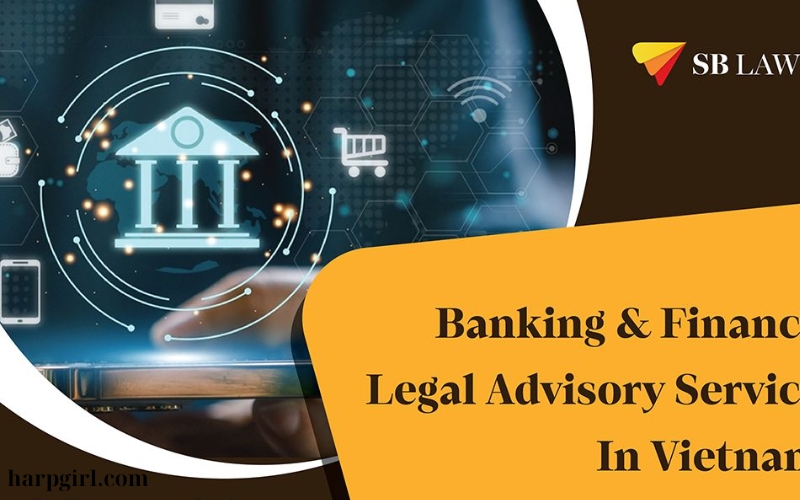
3.1. Healthcare: Transforming Diagnostics and Treatment
The healthcare industry stands to benefit tremendously from AI and ML technologies. Machine learning models are being used to analyze medical images, detect patterns in patient data, and assist in diagnosing diseases at an early stage. These AI-powered systems can detect anomalies in radiology scans, identify tumors in medical images, and even predict the likelihood of diseases such as cancer, diabetes, and heart disease.
AI also plays a pivotal role in drug discovery. By analyzing vast amounts of biological and chemical data, AI can predict the efficacy of potential drugs and even suggest novel drug combinations. This accelerates the drug development process, reducing the time and cost involved in bringing new medications to market.
Additionally, AI and ML-driven tools are revolutionizing personalized medicine by tailoring treatment plans to individual patients, improving outcomes, and minimizing adverse effects.
3.2. Finance: Enhancing Decision-Making and Risk Management
The finance industry has embraced AI and ML to improve decision-making, optimize processes, and enhance customer experiences. AI-powered algorithms are widely used in trading, with systems able to analyze market data, recognize trends, and execute trades at speeds far beyond human capability.
Machine learning models are also used to detect fraud in real-time, identifying unusual patterns of behavior and flagging suspicious transactions before they occur. These tools have drastically improved the ability of financial institutions to safeguard customer assets and maintain regulatory compliance.
In addition, AI is helping banks and financial institutions offer personalized services. By analyzing customer data, AI-driven systems can recommend tailored products, such as loans, insurance, and investment opportunities, based on individual preferences and financial behavior.
3.3. Retail: Revolutionizing Customer Experience and Supply Chain
AI and ML have revolutionized the retail industry by providing personalized experiences for customers. By analyzing consumer behavior, preferences, and purchase history, AI algorithms can recommend products, offer personalized discounts, and create targeted marketing campaigns. This level of personalization helps retailers build stronger customer relationships and increase sales.
Machine learning is also transforming supply chain management by improving inventory forecasting, optimizing delivery routes, and minimizing waste. AI-driven systems can predict demand fluctuations and adjust production schedules accordingly, improving efficiency and reducing costs.
3.4. Autonomous Vehicles: Redefining Transportation
One of the most exciting applications of AI and ML is in the development of autonomous vehicles. Self-driving cars rely on AI algorithms to analyze real-time data from sensors, cameras, and radar to navigate roads safely and efficiently. Machine learning models continuously improve the vehicle’s performance by learning from vast amounts of driving data, enhancing decision-making capabilities and safety features.
The widespread adoption of autonomous vehicles has the potential to reduce traffic accidents, improve fuel efficiency, and revolutionize the way we think about transportation. AI-driven systems also enable smart traffic management, reducing congestion and improving the flow of traffic in urban areas.
4. Challenges and Ethical Considerations
4.1. Data Privacy and Security
One of the primary concerns associated with AI and ML is data privacy and security. Both technologies rely on vast amounts of data to train algorithms, and as more personal and sensitive information is collected, there are increased risks of data breaches and unauthorized access.
Organizations must ensure that data collection and usage comply with strict privacy regulations, such as the GDPR (General Data Protection Regulation) in the European Union, and that AI models are designed to prioritize data protection. Ethical AI practices, including transparency, accountability, and fairness, must be at the forefront of AI development.
4.2. Job Displacement and Workforce Automation
Another significant challenge is the impact of AI and automation on jobs. As AI and machine learning systems become more capable, there is a growing concern that they will replace human workers in various industries. While AI can improve efficiency and reduce costs, it also has the potential to displace workers in sectors such as manufacturing, customer service, and data entry.
To address this challenge, governments and organizations need to invest in reskilling and upskilling programs that equip workers with the skills needed to thrive in an AI-driven world. This includes a focus on higher-level skills such as problem-solving, creativity, and emotional intelligence, which are less likely to be automated.
4.3. Bias and Fairness
AI and ML algorithms are only as good as the data they are trained on, and biased data can result in biased outcomes. If an AI system is trained on historical data that reflects societal inequalities, it may perpetuate those biases, leading to unfair or discriminatory decisions.
For example, in hiring algorithms, AI models may inadvertently favor certain demographics over others if the data used to train them is not representative. To mitigate these risks, developers must ensure that AI models are trained on diverse and unbiased datasets, and that they are regularly audited for fairness.
5. The Future: Opportunities and Innovations
5.1. AI-Driven Personalization and Human-Machine Collaboration
Looking forward, AI and ML will continue to drive innovation in personalization. From personalized healthcare treatments to tailored financial advice and shopping experiences, these technologies will enable even more customized and seamless interactions between consumers and businesses.
Furthermore, the future of AI lies in human-machine collaboration. While AI systems can perform tasks with remarkable speed and accuracy, human input and oversight will remain crucial for creativity, ethical decision-making, and complex problem-solving. The collaboration between AI and humans will lead to more efficient workflows, better decision-making, and improved outcomes across all industries.
5.2. Advances in General AI and Consciousness
As AI continues to evolve, there is the potential for the development of Artificial General Intelligence (AGI)—machines that possess the ability to understand and perform any intellectual task that a human being can. While AGI remains a distant goal, advancements in machine learning and neural networks may pave the way for machines that can learn and adapt across diverse contexts, much like humans do.
However, with this potential comes significant ethical and safety concerns. Researchers are exploring ways to ensure that AGI development proceeds with caution and responsibility, prioritizing human welfare and ensuring that AI systems are aligned with human values.